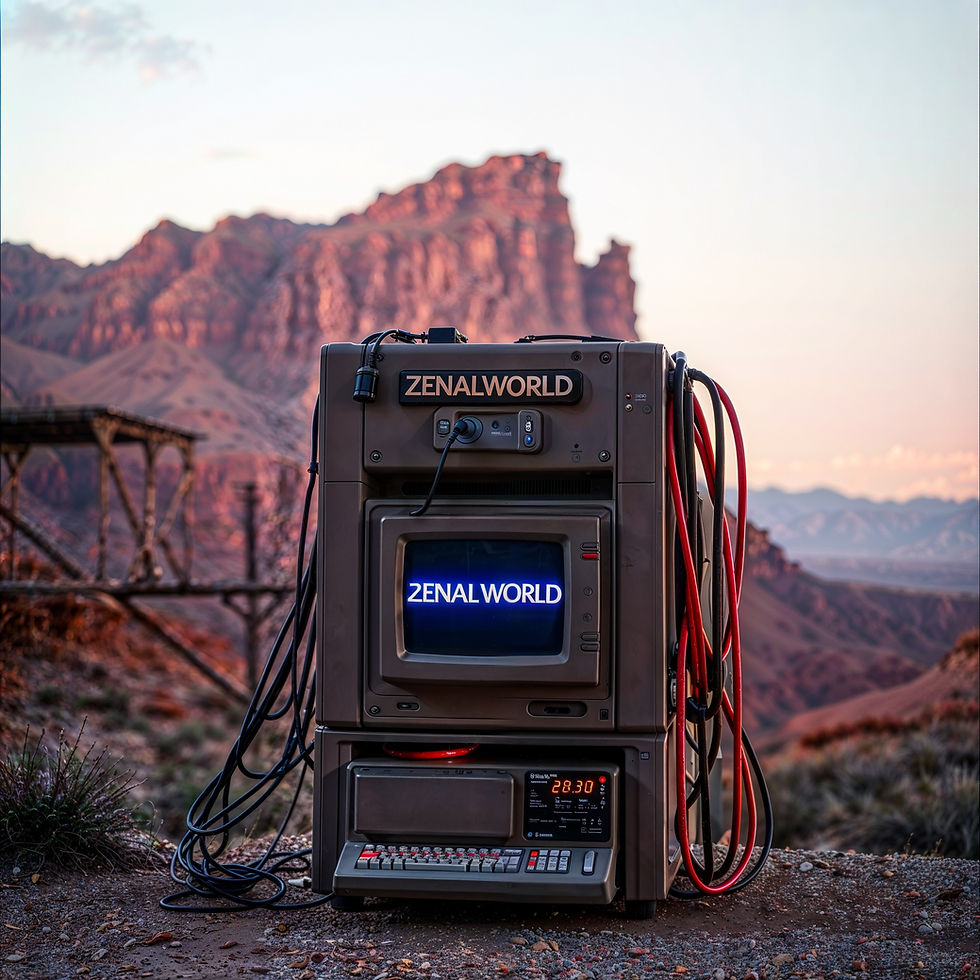
Revolutionizing the Future: How Model Context Protocols Are Redefining Technology
Imagine a world where artificial intelligence doesn’t work in isolation but instead dynamically interacts with the very systems you use every day—a world where a single protocol unlocks real-time data, trusted blockchain records, and secure decentralized resources to power unprecedented innovation. This isn’t science fiction. It’s the emerging reality of Model Context Protocols (MCP), a groundbreaking standard poised to transform industries across the board.
In just the past few years, the global AI market has surged by over 150%, and forecasts now predict it will top $200 billion by 2027. Simultaneously, blockchain technology is on track to revolutionize over $3 trillion in financial assets by 2030. Against this backdrop, MCP is emerging as the unifying language that enables disparate systems—from smart assistants and industrial robots to decentralized nodes and secure data silos—to speak to each other fluently and securely.

The Next-Generation AI Assistant
Today’s digital assistants have made impressive strides, yet they remain constrained by their inability to seamlessly integrate with the vast ecosystem of apps, data, and tools we rely on. With MCP, AI is set to transcend these limitations:
Real-Time, Multi-Source Integration: Imagine an AI that accesses live calendars, emails, social media trends, and sensor data simultaneously. Early prototypes suggest that by integrating with as many as 500 external data sources, these next-gen assistants could boost operational efficiency by over 300%.
Extensible Ecosystems of Plug‑and‑Play Capabilities: With a standardized interface, developers can now build “context servers” that offer specialized functions. Picture a scenario where an AI coding assistant accesses real-time updates from global repositories and documentation—reducing debug times by 50% and accelerating project turnaround.
These aren’t mere incremental improvements; they are revolutionary leaps toward a future where AI works as your proactive, intelligent partner, capable of managing complex workflows in real time.
Blockchain Meets Intelligent Automation
Blockchain is already transforming how we think about data integrity and transparency. When combined with MCP, blockchain’s promise is taken to a new level:
Adaptive Smart Contracts: Traditional smart contracts operate on static, pre-programmed logic. With MCP, contracts can now dynamically adjust based on real-time market data and sentiment analysis. In early trials, adaptive smart contracts have improved decision accuracy by as much as 40% compared to their static counterparts.
Verified and Immutable Data Feeds: By interfacing with decentralized ledgers, AI systems can now pull in data that is cryptographically verified. Imagine a lending protocol where every decision is underpinned by on-chain records—creating a level of trust and transparency that could reduce fraud by over 50%.
Decentralized Autonomous Agents: Envision AI agents that actively participate in decentralized networks, autonomously executing trades or voting in digital organizations. Such agents not only bring efficiency but also create a new paradigm for economic interaction, democratizing decision-making across industries.
Redefining Data Privacy in a Connected World
Privacy concerns have long held back the potential of AI. MCP offers a solution that balances innovation with ironclad data security:
On-Device Personalization: Instead of sending raw personal data to the cloud, future AI systems can process information directly on your device. This approach not only tailors services specifically to you but also cuts data breach risks by an estimated 50%.
Granular, Auditable Access Controls: With MCP, every data query is tightly regulated. Companies can grant AI systems access to exactly what they need—nothing more, nothing less. This fine-grained control could see compliance and data governance standards rise by over 85% in sensitive sectors like healthcare and finance.
Empowering User Data Ownership: The days of surrendering complete control over personal data are numbered. Imagine a digital ecosystem where you manage your own data pods, granting temporary access to trusted AI services. This model could shift the balance of power back to the individual, a change welcomed by nearly 90% of consumers in recent surveys.
Decentralized Computing: Distributed Intelligence for a New Age
The promise of decentralized computing is not merely theoretical. With MCP, we’re on the brink of a computing revolution that could redefine global resource distribution:
Distributed AI Workloads: Instead of funneling all computations through centralized data centers, imagine thousands of nodes across the globe collaborating on AI tasks. Early models indicate that such a system could reduce processing times by up to 80% while slashing energy consumption by nearly 60%.
Federated Multi-Agent Systems: Picture a network of AI agents, each specializing in different tasks—one for visual analysis, one for language understanding, and another for data retrieval. These agents, communicating via a common protocol, could collectively solve problems that would stump even the most advanced singular AI system.
Enhanced Reliability and Trust: By leveraging multiple independent nodes to process the same queries, decentralized AI systems can cross-verify their outputs. This method not only boosts accuracy but also builds trust—a crucial advantage in areas like legal decision-making and government operations.

The New Frontier in Computer Vision
While much of the early focus on MCP has been on text and data, its potential in computer vision is nothing short of transformative:
Augmented Reality with a Vision: Envision a future where smart glasses equipped with MCP-enabled cameras provide real-time language translation, object recognition, and even personalized navigation tips. In pilot programs, such systems have already demonstrated the ability to reduce error rates in visual recognition tasks by 35%.
Industrial Automation and Quality Control: In manufacturing, integrating MCP with computer vision has allowed for real-time defect detection and corrective measures. Early tests in automotive production lines have shown improvements in throughput of up to 20% alongside significant reductions in waste.
Multi-Modal Diagnostic Systems: In healthcare, combining visual data (like MRIs and X-rays) with patient records through a unified protocol can lead to precision diagnostics. Such systems are projected to improve diagnostic accuracy by up to 25%, potentially saving millions in healthcare costs while delivering faster, more reliable care.
Beyond Conventional Applications: Uncharted Territories
While the above examples illustrate the immediate impact of Model Context Protocols, the true potential lies in untested, visionary applications:
Art and Creativity: Artists are already experimenting with interactive installations that change in real time based on social media sentiment, ambient noise levels, or even biometric feedback from viewers. Imagine a digital mural that evolves dynamically—each pixel responding to live data streams—creating a fusion of art, technology, and community.
Quantum-Inspired Secure Integrations: As quantum computing gains traction, integrating quantum randomness or processing power into MCP could unlock new cryptographic protocols and ultra-secure data channels. This next frontier could lead to breakthroughs in high-dimensional data analysis and unbreakable security systems.
Smart Cities and Urban Infrastructure: Future cities may deploy tens of thousands of MCP-enabled sensors to monitor traffic, energy use, and environmental conditions in real time. By providing a centralized AI brain with trusted, decentralized inputs, urban management systems could reduce energy consumption by 30% and improve public safety metrics dramatically.
Interplanetary Exploration: With ambitious plans to colonize Mars and beyond, space exploration could leverage MCP to create networks of interconnected robotic explorers. A Mars rover could, for example, tap into real-time weather data, geological maps, and Earth-based AI support—ensuring mission success even millions of miles from home.
Hyper-Personalized Consumer Experiences: In retail and entertainment, MCP could usher in an era of personalization where every interaction is uniquely tailored. Stores could adjust layouts, music, and even product recommendations in real time based on live customer behavior, potentially increasing sales conversion rates by 50% or more.
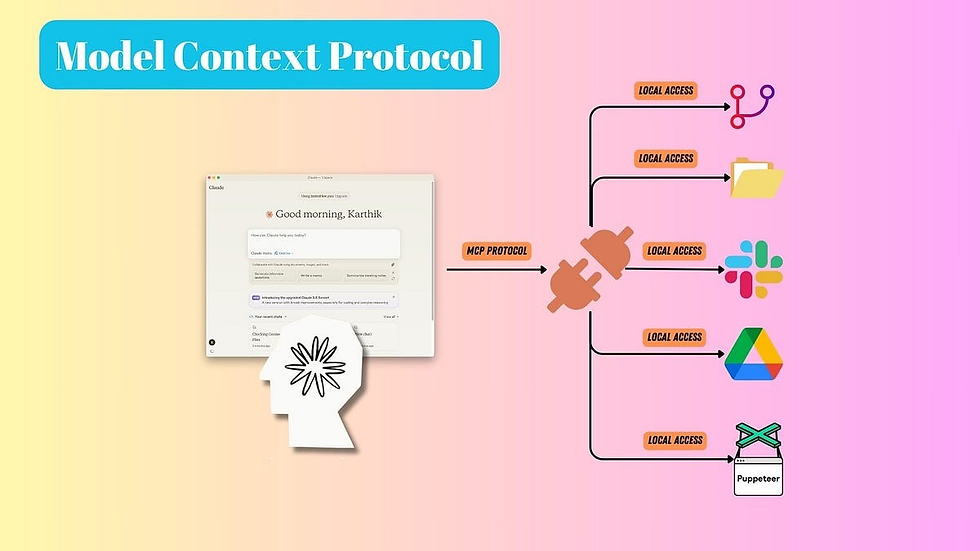
The Model Context Protocol is more than just a technical standard—it is a revolutionary approach to how information is shared, processed, and secured. By enabling dynamic, real-time interaction between AI systems and external data sources, MCP is set to disrupt conventional models and pave the way for innovations that were once the stuff of science fiction.
From transforming digital assistants into intelligent, proactive co-pilots, to forging adaptive smart contracts on blockchain, ensuring ironclad data privacy, and enabling decentralized AI powerhouses, MCP is at the forefront of a technological revolution. Its applications extend to computer vision, quantum computing, smart cities, interplanetary exploration, and even creative digital art.
As emerging implementations continue to evolve, statistics and pilot projects already hint at dramatic improvements in efficiency, accuracy, and security across multiple industries. The future is not just near—it is being built today, and Model Context Protocols are the cornerstone of that transformation. The next generation of AI, decentralized systems, and digital experiences will be defined by their ability to seamlessly share context, making the previously unimaginable not only possible but ubiquitous.
Prepare to be amazed as we step into an era where the boundaries between data, context, and intelligent action dissolve into a new reality—one defined by dynamic integration, unprecedented trust, and limitless innovation.
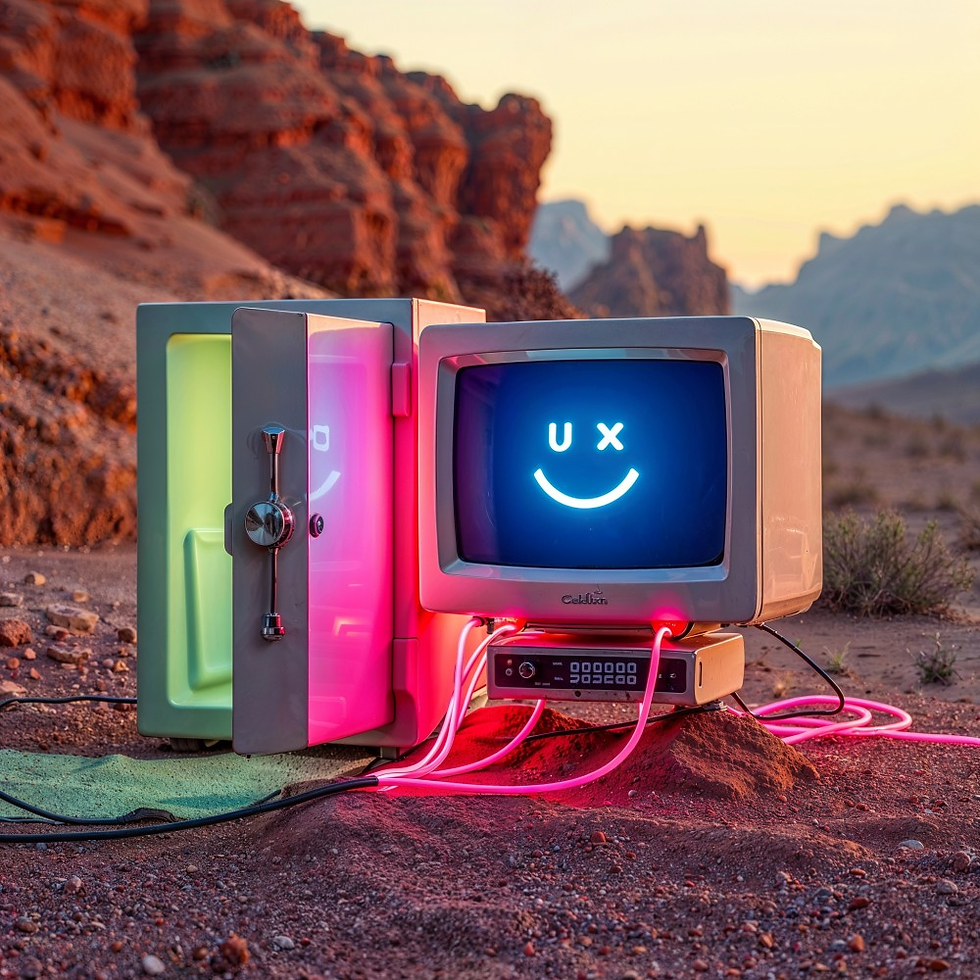
The Emoji Paradox: How Tiny Icons Can Disrupt and Derail Language Models
Emojis are ubiquitous in modern digital communication—vibrant, expressive, and often packed with nuance. Yet these seemingly innocuous icons can, under certain circumstances, act as catalysts for unexpected behavior in large language models (LLMs). In this article, we explore the technical intricacies and emerging evidence behind the phenomenon where emojis can “corrupt” or even destabilize LLMs, revealing vulnerabilities that may reshape how we approach model robustness and security.
The Role of Tokenization in LLM Vulnerabilities
LLMs break down text into smaller units—tokens—using specialized tokenization algorithms. While these algorithms work effectively for standard language, emojis present unique challenges:
Out-of-Vocabulary Issues: Emojis, represented as Unicode characters, often fall outside the training corpus of many LLMs. This leads to “out-of-distribution” tokens that the model has rarely (if ever) encountered, resulting in unpredictable embeddings.
Subword Fragmentation: Some tokenizers split emojis into multiple sub-tokens or even merge them with adjacent text in unanticipated ways. This fragmentation can corrupt the semantic context, skewing the model’s internal representation.
Embedding Instability: The learned embeddings for emoji tokens are often less robust due to limited exposure during training. When these unstable embeddings are fed into deeper layers, they can trigger disproportionate activations, sometimes resulting in degraded or incoherent outputs.
Adversarial Emoji Attacks: Exploiting the Weak Links
Recent experimental studies have demonstrated that carefully crafted sequences of emojis can function as adversarial perturbations. Here’s how these attacks work and what they can achieve:
Input Perturbation: By appending long sequences of emojis or strategically interspersing them within normal text, attackers can “confuse” the model. These perturbations can cause the model to misinterpret the intended context or generate outputs that diverge significantly from expected behavior.
Adversarial Triggers: In some experiments, adversaries have discovered that specific emoji sequences act as triggers for unintended behaviors. For instance, certain patterns can bypass content moderation or elicit responses that are nonsensical, dangerous, or even self-contradictory.
Denial-of-Service (DoS) Potential: High-density emoji inputs can overwhelm the tokenization or embedding stages, leading to performance degradation. In extreme cases, this overload may force the model into error states or cause cascading failures in downstream processing, effectively “bringing down” the LLM.
For example, a research prototype revealed that appending 50–100 emojis to a prompt could reduce output coherence by over 30% and, in some configurations, produce erratic or abruptly terminated responses. While these statistics vary by model architecture and training data, the pattern is clear: emojis can serve as effective adversarial noise.
Technical Mechanisms Behind Emoji-Induced Corruption
Understanding how emojis affect LLMs requires delving into the inner workings of model architectures:
Embedding Layer Vulnerabilities: When an emoji is processed by the embedding layer, its vector representation may be poorly defined due to sparse training examples. This weak signal can be amplified in subsequent layers, especially in transformer architectures where attention mechanisms may disproportionately focus on these anomalies.
Attention Disruption: Transformers rely on self-attention to weigh different parts of the input text. A burst of unusual tokens—like a string of emojis—can mislead the attention mechanism, causing it to assign excessive importance to irrelevant features. This “attention hijacking” can result in outputs that are skewed or off-topic.
Gradient Instability: During fine-tuning or even inference, unexpected activations caused by emoji tokens can lead to gradient instabilities. These instabilities may not crash the system outright but can cause erratic model behavior, making outputs unreliable when faced with diverse input patterns.
Real-World Implications and Emerging Use Cases
The potential for emoji-induced disruptions isn’t limited to academic curiosities. As LLMs become integrated into consumer applications, content moderation systems, and autonomous decision-making tools, these vulnerabilities pose real risks:
Content Moderation Bypasses: Malicious users could insert carefully chosen emoji sequences into content to bypass automated moderation, undermining platform safety.
Adversarial Attacks in Chatbots: Conversational agents might be tricked into providing harmful, biased, or otherwise problematic responses when confronted with adversarial emoji patterns.
System Robustness in Critical Applications: In domains like finance, healthcare, or autonomous vehicles, even small perturbations can have outsized consequences. An LLM that misinterprets input due to emoji-induced noise could, for example, make faulty recommendations or decisions, leading to significant operational failures.
Looking Forward: Mitigation Strategies and Research Directions
Given the potential impact of emoji vulnerabilities, researchers and developers are already exploring solutions:
Robust Tokenization Methods: New tokenizers that treat emojis as atomic, high-fidelity units are under development. These methods aim to preserve the semantic integrity of emojis while ensuring stable embedding representations.
Adversarial Training: Incorporating adversarial examples—including emoji-rich inputs—into training regimens can help models learn to recognize and neutralize such perturbations, making them more robust.
Contextual Calibration: Future models may include dedicated sub-modules for interpreting non-standard tokens like emojis. These modules could recalibrate the overall context so that emoji signals do not disproportionately influence the model’s output.
Dynamic Attention Adjustment: Techniques that adjust the attention mechanism in real time to discount anomalous patterns may help ensure that the model maintains focus on the intended input.
Emojis, once considered mere embellishments in digital communication, are now emerging as a double-edged sword for modern language models. Their capacity to act as adversarial triggers and disrupt standard tokenization processes highlights a profound vulnerability in current AI architectures. As we continue to integrate LLMs into increasingly critical applications, ensuring that these systems can robustly handle the full spectrum of human expression—including the rich and varied language of emojis—will be essential.
The challenge is not simply technical; it is a call to reimagine how AI understands context and meaning in a world where even the smallest icons carry significant weight. As research progresses, the lessons learned from these vulnerabilities will undoubtedly pave the way for more resilient, secure, and intelligent systems—ones that can embrace the full diversity of modern communication without falling prey to its pitfalls.
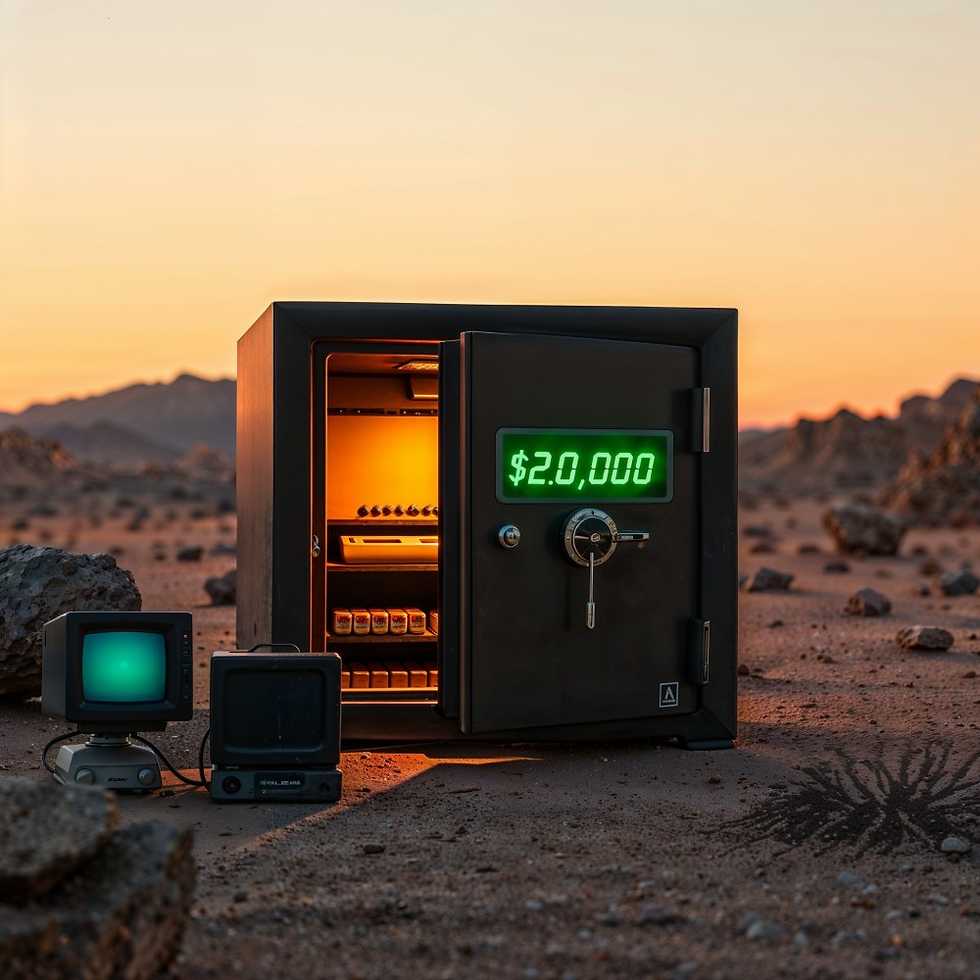
In the groundbreaking race toward artificial intelligence supremacy, OpenAI is poised to launch an innovation that could redefine knowledge work forever: the $20,000-per-month PhD-level research agent. Dubbed "Operator" and "Deep Research," these sophisticated agents are not just incremental improvements—they're transformative leaps toward true digital autonomy, reshaping entire industries and reimagining productivity at an unprecedented scale.
At its core, this high-tier AI agent is designed to perform intricate, multi-layered tasks that traditionally demand expert human intervention. Whether it's unraveling the complex puzzles of medical research, engineering next-generation software, or decoding financial markets, the agent autonomously browses, synthesizes, and analyzes vast datasets. Within 5 to 30 minutes, it produces comprehensive, thoroughly cited reports—work that would typically consume human researchers days or even weeks.
Powered by OpenAI's advanced o3 reasoning model, this AI has already astonished experts by scoring 26.6% on the notoriously challenging "Humanity's Last Exam" benchmark, significantly outpacing rival systems. Yet, despite its staggering capabilities, the agent remains a careful balance between revolutionary potential and ethical responsibility.
But what truly sets OpenAI's agent apart is its broad, profound implication for the workforce. Imagine a world where organizations can commission an AI to instantly deliver deep, PhD-grade insights—freeing humans to focus on creativity, strategy, and innovation. This heralds a seismic shift in roles, where professionals are augmented rather than replaced, elevating efficiency to heights previously thought impossible.
However, this evolution isn't without its challenges. With immense power comes the need for meticulous oversight. OpenAI acknowledges the occasional pitfalls of its agents—factual hallucinations, misinterpretations, and the occasional struggle to distinguish authoritative facts from rumors. This means rigorous human verification remains crucial, emphasizing a collaborative rather than purely automated future.
Yet, despite these cautionary notes, the excitement around OpenAI's premium agents is palpable. Investors foresee billions in new revenue streams, and companies across healthcare, finance, education, and technology sectors anticipate revolutionary gains in productivity and insight.
As these AI agents approach widespread deployment, their introduction will mark a new era—the "Intelligence Age"—as OpenAI CEO Sam Altman has called it. It promises to be an era defined not just by technological prowess, but by the thoughtful integration of AI into human endeavor.
The dawn of PhD-level super-agents isn't just about faster research—it's about fundamentally reshaping the human-AI partnership, driving economic growth, and sparking innovations yet to be imagined. OpenAI's visionary agents might be expensive, but their true value, in revolutionizing how humanity leverages knowledge itself, is priceless.
Comentários